In an extra-special webinar, Neuroscientist turned marketer, Giulia Panozzo, delivered a workshop on SEO testing for us.
Find out how to run tests for SEO step-by-step, from formulating your hypothesis to presenting your data to stakeholders. You can expect to learn:
- How to prioritise test ideas
- How to choose your test design and timeframe
- Selecting test and control groups
- Extracting and analysing data
- Data visualisation and storytelling
We recommend watching this and working through Giulia's slides here.
Watch the webinar below
Subscribe to the Sitebulb YouTube channel! You can also sign up here to be the first to find out about upcoming webinars.
Webinar transcript
Jojo:
Hello everyone. Welcome to this extra special Sitebulb webinar. We've got a workshop with Giulia Panozzo. I really hope I said that okay. On SEO testing. Please say hello in the chat and tell us where you're from. So I'm Jojo, I'm Sitebulb's marketing manager. And for those of you who don't know, Sitebulb is a website crawler and auditing tool for technical SEO. So feel free to look us up. We have a desktop and a cloud version of Sitebulb. So whatever kind of SEO you are, there's a Sitebulb plan to suit. I'd like to welcome our awesome trainer, Giulia, who is a neuroscientist turned marketer, independent researcher and advisor, and international keynote speaker and author. So we're extremely lucky to have Giulia presenting this workshop for us today. The session is being recorded, so don't worry if you miss anything, I'll email out a link to the recording tomorrow. We should hopefully have a bit of time at the end of the session today for your questions.
So please put them into the Q&A tab, which is next to the chat. Don't put them in the chat box itself ideally. And then you can also upvote other people's questions in there if you can't think of any yourself. Okay, let's get this workshop started. I'm going to head off now, and I'll see you later on for the Q&A. Don't forget to get your questions in. Giulia, thank you so much for doing this. And over to you.
Giulia Panozzo:
Thank you so much Jojo for the lovely introduction. And thank you all for joining. So my name is Giulia Panozzo, as Jojo introduced, I'm an in-house director of customer acquisition for a global marketplace, but I'm also a freelance consultant, especially on the topics of neuromarketing, because my background is in neuroscience. And coming from an academic background, I love testing. Even when I went into SEO, that's an element that I retained in everything in my strategy. And as a part of that, I've been part of teams where testing is not really prioritised in the budget. So there's never enough money to unlock tools, for example, or unlock the testing mindset. So I had to do a lot of it on my own learning to use free tools. So today we're going to go through some of my process with free tools, with freemium tools so that you can start testing on your own.
Just as a disclaimer, this deck is massively nineties coded, so I hope there's some millennials in here which are going to be appreciating the nineties references, because there's a lot of information, but I also wanted to make it a bit more fun. So first of all, what is SEO testing? SEO testing is when we investigate the effect of SEO changes on a variable of interest. These can be clicks, that can be rankings, that can be page speed, anything that you consider to be a metric of interest for your own business. But why do we bother testing? So I want to leave it to the chat for a few seconds. Why do you think it's important to run tests on your website? Is it to inform the SEO strategy? Is it to figure out what works best for your audience? Is it to avoid SEO disasters, or is it to show stakeholders the importance of SEO? So I'm going to leave it to the chat.
To be honest, I cannot see the chat, so I'm going to rely on Jojo to let me know. But yeah, does anybody have any guesses? Jojo, is there anything in the chat? Well, if I don't know what the guesses were, but-
Jojo:
All of the above we're saying, everyone is keen to vote all of the above.
Giulia Panozzo:
Awesome. So yes, all of the above. Exactly. So there's another reason why I like to use it, which is to settle a tech debate. And that's because I'm not really great with assertiveness. Sometimes I find solace in data. For example, one thing that a lot of SEOs will probably resonate with is during migrations when you might have to make a plan for moving URLs to another location and maybe best practises are not enough to make your case. So I had a situation, for example, where recommendations from a client were different from mine, and essentially I had to make a decision based on data, because they were planning to migrate everything to non-indexable URLs, which obviously we know that is not great. So one thing that we can do to settle this is like, okay, can we test it? And then we see what actually brings the best results. So today we're going to see the process across these five steps.
So we're going to embark on a journey to see how to formulate an hypothesis to test, how to select the test design, how to choose the test and control groups, how to do data extraction and analysis, and then reporting. Again, this is my process. You might have a different process based on what your tools available are, but this is something that has worked consistently for me. So first of all, the hypothesis, what is it and how do you create one? A hypothesis is a statement that is normally made up of an independent variable and a dependent variable. So these are normally the two elements that you definitely need to create an hypothesis. In general, it's the change that's being tested. So that will be your independent variable. And then the expected outcome of that change. And that will be your dependent variable. You can be even more precise, you can establish by how much you expect the dependent variable to change, and also in what timeframe. But these are generally the minimum elements that you will need to create a hypothesis.
And how do you start formulating a hypothesis? It all depends on what you're trying to answer and what your personal day-to-day business struggles or challenges are, what stakeholders are asking about. So for example, you can look at your metrics. Is anything going down, for example. You shouldn't test just for the sake of testing, of course, but it's hard already to figure out how our strategy should be when there's so many algorithm updates and so many changes in the SERP. So prioritise based on what's most likely to make impact to the business. And you can take a look as well at what might need improvement based on your technical audits. And that's something that Sitebulb does really well. It gives you hints already on what might be some things that you want to focus about. So if you need any ideas, you can rely on the technical audits as well. So some of the example questions that might drive your testing strategy are based, for example, on maybe your traffic is down.
So how do you leverage, for example, your content to improve traffic? Is this something that can be best performing if it's coming directly from your customers? So UGC material versus default material from the perspective of the brand, or maybe it can be tests like testing longer form content versus short form content on pages, or AI-generated versus human content, rich text versus plain text and so on. There's so many options. Or will the navigation maybe in site architecture help us achieve better rankings and more clicks? So again, different metrics, different strategies, but these are also some useful pointers to create your hypothesis. Or what on-page elements are most likely to create an increase in CTRs, especially now with changes to the SERPs, we know that CTRs are generally dropping, especially when it comes to marketplaces and ecommerce. So what do we leverage in order to counteract those changes? Or do product reviews have an impact on PDP conversions at all? And this is not just out of curiosity. But testing can actually provide the case for a product feature that you might otherwise not be able to push for.
So if you need any inspiration, again, look at Sitebulb, look at the technical audits, but there's also these resources that are great, and that I've definitely used for my own testing ideas. And again, this is recorded, so you don't need to take screenshots for now. So moving on. The second step, once you have decided what your hypothesis is going to be is going to be selecting your test design. So there is three main test designs that I normally focus on. There's A/B testing or split testing, multivariate testing, or time-based testing that I call pre-post. And your hypothesis should already define what your design is going to be, because your questions will be able to inform what design is best suited to utilise in your test. So look at your variables, for example, specifically the metrics that you're looking to get an effect on and change that you want to implement.
Look at the tools that you have available. Are you able to leverage tools that do server-side testing, or is it going to be more on the client-side testing. And look at the existing traffic. Is your website big enough and with enough traffic to leverage bigger groups of URLs to test? Or on the other hand, is it a bit smaller, and maybe you might benefit more from a time-based test. So this is kind of a cheat sheet to understand a bit where the testing timeframe sits for each one of these design.
Giulia Panozzo:
A/B and multivariate testings are normally when we split, we don't split users in SEO testing, we split pages, but we test them across the same timeframe. So I implement the change, for example today, and then I check across the two groups, which one performed best in two weeks or four weeks. And then the traffic required for these ones. A/B testings resides normally on the low to medium spectrum, whereas multivariate requires a bit more traffic to obtain a bit more results that are reliable. Again, as we mentioned, for small websites that have a bit less traffic, a time-based testing. So a pre-post test might be more suited. That's when you implement the change and then you compare the outcome to the previous performance. So let's see a few examples. So A/B testing, for example., The core principle of A/B testing is to isolate and test a single variable at a time while keeping other factors constant. We can see here the content placement has changed between the two variants, A and B. So A/B testing we mentioned for SEO is a bit different from CRO and UX, because you're not splitting users, you're splitting pages.
So if we go back to the nineties and take some examples to make this a bit more relevant. Say that you are a music producer and you want to see which one of these albums goes down with the audience better. Actually the same day was the release day for Blood Sugar Sex Magik by Red Hot Chilli Peppers and Nirvana's Nevermind. So great example of A/B testing. Two different albums that went out on the same day. And if we were to compare in the same timeframe, I could identify a clear winner in Nevermind.
So let's see the multivariate testing instead. This is sort of an extension of the A/B testing when we have multiple changes going on at the same time. So you can see here, not only the content placement has changed, but also the content format. So I have normal content here, and then I have FAQ and then the placement changes in version C. Same thing, a third album was released actually in the same day. So The Low End Theory by A Tribe Called Quest, which I've not heard. But again, the winner stayed Nirvana. So when we look at time-based testing, instead of what I call pre and post, it's when I want to keep my tests simple. And this is for small websites, this is for a number of cases where it makes a lot more sense to use time-based rather than A/B testing.
And I focus a lot on these tests when I was at the start of my testing career, because you can still see very valuable results. So you can see here change the content, and I had a look at results in two subsequent timeframes. This is great for single on-page changes. The drawback is that you might need to take into account what goes on externally, obviously, because you don't have a lot of data to go on to go off from, so you need to take into account all of the external variables as well. It's also good for all of those testing groups that cannot be matched to a control group, or to another conditions, for a number of reasons. Maybe there are particular pages that just don't have a comparable control, or maybe the sample is too small, or the traffic is too low. And it's great for off-page campaigns as well, because we know that SEO doesn't rely only on on-page signals, but also off-page signals. And this is great for example, to establish the impact on search for a TV campaign launch for example.
Something that I came across when I was looking at this year collective, which is another marketplace, and it was mentioned in one of the episodes of Emily in Paris, and you can see the brand searches went up. So it's a great tool to figure out the cross-functional efforts and their impact on searches specifically. So once you select your hypothesis and your design, it's time for you to decide what pages are suitable to test, which can be quite challenging. So how do you select your test group? In general, I got my cheat sheet. And my good rule of thumbs that are you want to select pages with enough traffic. So not only like two to three clicks a day. Not your outliers, so don't pick your lowest end or higher end pages, because those will not be comparable to other pages. And also you want to compare pages of the same type. So for example, product listing pages.
You don't want to lump in product pages and product listing pages, because they will have different intent and different navigation structures. So just keep everything as consistent as you can. And this is all to help reach statistical significance, which you don't always need. But I think it's helpful when we need to make a case. Because again, like I find that data really allows me to make data informed decision and I trust it. I trust it much better than hunches. So how do you select the control group? So what you're going to test against. Keep everything as close as possible to the test group, because you want your control group to be comparable to your test group.
There's a few options that I normally use, and that can be defined by your business and your website. But if you have an international website, I think this is great, this first option. So you take the same page as the test, or same group of pages as the test groups, but in a different market where the change has not happened. So you treat one market and you compare it to another market that has not had the change. Obviously you need to take into account that you don't test during one of those markets holidays periods, because then you have an external variable that doesn't make the two comparable. But in general, this is a good one to use when you have more international [inaudible 00:16:08].
Another one that I use quite often is to control against the whole website trend. So you have your test group, and then you have a control that it's like the overall trend of the website. This might not work with smaller website, because maybe the test group will heavily influence your actual trend. But with big websites, checking against the general site performance helps detect those cases where the example, well, for example, there's a lack of impact because the trend is down across all of the pages. Or on the other side, it might even strengthen your case when you see that the test is going up, but the trend is going down. So you can see that the test actually has a great impact, while the general trend is going down.
And finally, you can use URLs that are randomised between control and test group that come from the same sample pool as a test group. So this is great for small scale website and tests. But you can also do it, because you can see in this screen this was all done manually and you want to control that they have roughly the same baseline traffic so that they're comparable. But if you have a bigger website, you can still do this, just use some help. So if you want to use ChatGPT or any other LLMs, you can do it. Just make sure that when you submit your file, you just change the name of the website, because you don't want to feed in your personal business information and deep data analytics into an LLM. But that can help. However, we did say that I was going to use free tools, and ChatGPT at some point didn't want to do any analysis for me, because I have been using it a bit too much.
So there's other randomizers that you can use online. But personally I like to use RStudio. And bear in mind that you can, this is the way that I do it. This is something that makes it quite easy for me to randomise, but you can also use CoLab and Python, et cetera. And again, these resources can be found at the end of the presentation as well. So as long as you follow. Now you don't need to take a lot of notes. So the way that I randomise the groups between test and control groups on RStudio is by obtaining the data that I want to match by URLs first. So for example, I want to match by traffic for the last 12 months. I want my groups to have comparable traffic. So I'll need to obtain the click data from Google Search Console, for example, or anything else that might be the source of truth for your own website. Then I input my raw file that you can see here.
So this is like when I tell RStudio to read my file. And then I input some formulas that essentially just say bring back the two groups. It defines their size that I want them to be the same size, and I also want them to have the same amount of clicks, so that they are comparable for testing. And then I input these two commands that bring them back in the environment. But then again, you can see here I had 490 more rows, so I had a group that was 500 test URLs and 500 control groups. Then I might want to use this other command which brings them back directly in a CSV file. So this is the way that I randomise. And I think that's one that I found to be quite reliable as well. So you have your two groups, you run the test based on the changes that you want to make. But how long should you run it for? I know that a lot of people have been asking me this in several iterations of this webinar or like this masterclass. I would say it depends.
I know that this is something that pains a lot of SEOs to say. But it depends on what your goals are, what your traffic is. I normally recommend between two and four weeks is enough to see a trend within intermediate check-ins. And the reasons why I put intermediate check-ins there is because if there's something that goes extremely wrong, then you can spot it easily, and you can revert the test before. So when I look at my pre and post-periods, this is specifically for when I look obviously at my performance time-based, but also between groups. Your launch date is what informs the pre-period and the post-period. So what you're going to compare. I normally say for the launch date, because that's been a topic of debate as well, for SEO tests, just rely on when the change has been crawled rather than implemented.
So not only when you launched the change but when it's actually being seen by Google. And that's something that you can do with CyPulse as well. You can use the custom extraction function to verify this. And that's actually quite easy, because you don't even need to find the XPath or anything. You can just input your page, and then click on the element that you want to extract. So for example, say that your test focuses on updating the H1 on a series of pages. So you go from garden benches to garden benches and arbours. You can ask CyPulse to extract the H1 of all of the pages that you're testing to verify that it is definitely the new version that they're seeing. So yeah, that's quite helpful from CyPulse as well. Okay, I hope you're still with me. I know that it's a lot of content, and we still have a bit to go. But we made it to part four of five, so we're already quite ahead.
So the fourth step in this, once you have done your hypotheses, you selected your test design, then you have done your tests and control groups and everything, then you have to extract and analyse your data. So tools that I use quite a lot are these ones. And test design resources will determine what you use as well. So if you have any other tools then you're free to use anything. But I found for example, Google Search Console for me is quite easy to extract data from, especially if I use the search analytics for tools extension. So let's walk through an actual example on how to extract and analyse data when I have an actual A/B test that is running. So say that you want to test what content is better on PLP pages. So on product listing pages.
I took Argos as an example. I don't work for Argos, never worked for Argos. So this is just like how to make an example, because they don't currently have on these pages any content. So in my test I decide to include content in here. And again, I have one version that will have AI generated content and one version that will have human content. The conditions of the two groups should be the same. So make sure that you have similar baseline traffic per group that you can get by Google Search Console, and similar equity and architecture that you can see via Sitebulb. When I say similar structure and architecture, or like similar equity, it's because you want to make sure that there are pages that sit on the same level of your navigation, so you don't compare maybe stuff that sits on level zero and level one, but I want to extract in this case everything that resides under the garden and furniture page, or under the garden and furniture subfolder.
And what I do is also I decide to take away anything that has paginations, because then my dataset is much cleaner. So by using the inclusion and exclusion rules on Sitebulb, I can also bring back this file that allows me to see all of the URLs that can be my A and B groups that reside under the same structure under garden and furniture. So I know that it comes from the same place and it's comparable. And then I export this, I run my test and everything, and I decided my metric of interest, well before I run the test, I decided my metric of interest is going to be clicks and average position. Again, your metric of interest is your dependent variable, and you can choose it to be whatever makes most sense to you. This is just out of making an example that makes sense for me. My hypothesis is that group B will have higher clicks and better average position as group A. So I expect the human content to perform better than the AI-generated content.
Then I run my test, and then we wait for a bit. And then this is when we start to extract and analyse data. As I mentioned, I like to use search analytics for Sheets, which is a free extension. It kind of feeds in from your Google Search Console data. So you need to have the verification on your Google Search Console account. And it will bring back 16 months worth of data. You can always filter the dates to make most sense for you. And then what I do is I group by date, because I want to see the performance by date. I also want to filter by the pages that I run the test on. This ensures that not only that you capture only exactly the URLs that you run the test on, because otherwise it brings back a lot of data that you might not need of course. And when you group by date, you cannot really see what pages are included.
And then I request the data. But let's take a step back, because you've seen me inputting regex here. I know that other people say regex, I still don't know what's better to say, what's best to say. But yeah, so how do I get there? How do I get to the regex to define specifically what URLs I want to bring back from this extension? So this is my process in general. I find the identifier within each of the URLs that I want to bring back. So you can see that I have group A, group B, group A, group B, so these are all of my groups, my two groups. And this is the export that I got back from Sitebulb when I did the inclusion rules to bring back anything that was under the garden and furniture subfolder. So you can see that the identifier for these categories is normally here after the C. So that's my regex. You can see a number of symbols here.
I'm sure that a few of you will already be quite familiar with this regex, but essentially we're going to see it in a second. This part is what brings back everything that is before the C. The dollar sign defines the end of the input, so that I only bring back that page specifically and not anything that matches partially the input. So you can use whatever makes most sense for you. And then you can concatenate to obtain a single regex formula. But that can become quite taxing if you have a lot of URLs. So I use blind text to help myself with that, which is a text editor and makes it a lot quicker, because anything that you have in this Excel file can just go into different rows, and then you can bring it back into the same one. So these are some of the, I think the essential rules that you need to know, essential tokens.
The alternatives. So this establishes the regex needs to match either this formula or this other one. The catchall which we defined before, it brings back anything that either is before the regex or after. The dollar sign asserts the ends of the input. And then there's the escape characters, which is for special characters when you need to include them. For example, when you have a column that needs to be there, then it is part of the characters to be escaped rather than part of the formula itself. But anyway, there's a lot of resources on this one as well, and always validate via regex101. This is my best friend whenever I need to run regex. You can see in this case I always validate and my formula matches only part of the data set, which in this case is expected, because I established the end of the input. So I know that I'm not going to have any other subcategories that might originate from the same URL.
But you can see here, for example, there's other stuff after the category identifier. And this is no match, because I input the dollar sign which says, "No, you need to match up until here and nothing else." So again, further reading and resources is here, why regex is quite good when you need to extract data. So now that you establish your regex and have validated that it's going to bring back only the pages of interest, you can request the data on the extension as we've seen. And this is likely the output that you're going to have. So by date with clicks and position in two different columns. There's one more step than I normally do, which is to ensure that the data I obtain is definitely only coming from the pages I selected. So we've seen that before I group by date to obtain this kind of output.
But when I group by page, this allows me to ensure that all of the pages that I intend to analyse are included. Because it happened to me that maybe I run the first grouping and everything looks fine, and then I check a grouping by page that all of my pages are there, and I notice that there's pages that are not there. So I go back and look what the problem might be. And I notice that, for example, one of the pages that I'm testing on is no index. So there's no data that comes up from that. So yeah, essentially you run these two steps. One is to validate your output, and one is the actual one that you're actually going to analyse. So when I have my export by date for both groups, then I'm at the point where I'm happy, and in a position to compare. And your output is going to be likely like this. So yeah, this is like a disclaimer that I want to do at this point. You can test with anything, but always make sure that the conditions are the same.
And for example, this is something that I noticed when I run the second part of my validation system when I run by page, and I noticed that some of these pages were not coming through. And that was, because maybe the robots were not the same as the test group. So this is a point where some apparent errors will come out if you have anything that doesn't match conditions with the test group. So where are the parts of analysing? So if you have any budget to assign to tools, this is a great set of tools that you can use as well that makes the analysis really easy. If not though, you can always rely on delta formulas, which are quite easy to use, because it just compares the post data versus the pre-data and then runs a percentage on it. So you can see here, I think if, yeah, this is a GIF, so it should trigger and show you how I normally do this.
So you can see here I got my pre and post data, and then the delta is 27%, 0.7% of an increase in clicks across the 28 days. So this is a good starting point. However, another thing that you can do, especially for time-based analysis is the Causal Impact Analysis. So I did write a guide, a step-by-step guide, on causal impact on the women in tech SEO resources. So go and find that one, because I'm going to fly through these slides just to show you what causal impact can do and how powerful it is. But the step-by-step is much easier to understand if you have a guide under your eyes. So just to expand a little bit on causal impact, it's a powerful package to analyse data that infers the cumulative impact of a change. So for example, anything that you use as a treatment in a time series, and it's based on a statistical model called Bayesian Structural Time Series. It uses the past data to predict the outcome in the absence of the treatment.
And this predicted outcome is called the counterfactual. And then it takes the counterfactual and defines the impact based on its deviation from the actual outcome. So we can go back and look at it more in detail. But essentially it takes any input that might look like this after a test, takes the pre-implementation data, the post-implementation data, and spits out something that is looking like this. So it's much easier to appreciate if we compare it to the previous graph. And if we look at the singular components of causal impact, the full black line is your actual outcome. So this is for example, your clicks after the change. This is when the launch date, when the change happened, the dotted vertical line. And this is what your clicks look like after.
The second line, the dotted line is the predicted outcome. So this is essentially the counterfactual. The prediction of clicks if you had never put the change in place based on the previous performance. And then in the second panel you have the deviation and the cumulative impact as well. So causal impact is great, because it gives you the confidence to leverage statistically significant results and write changes at scale. So it tells you exactly by how much the change impacted the variable of interest. And it allows you to take out the guesswork, which we know as SEOs we really want to be data informed. You can use it on almost any time series data. So if you have an A/B test, it doesn't mean that you cannot use causal impact, because you have two groups and you might be able to run causal impact for both of them across the time series that you're looking at. There's this example from the Barbie movie similar to what we've noticed before, but this is a good one. Causal impact is a good one as well to look at the impact of PR campaigns. This is the Birkenstock category.
These are the Birkenstock searches after the premiere of Barbie movie and the trailer. So you can really take some great data out of it. And the reason why I really like to use it is because by identifying clearly and not just out of a hunch, if something is a winner or a loser for our audience, you can literally figure out and nimble out the work that you want to do next. So there is, again, this is something that's much easier probably in the guide, but I'm going to show you a tiny demo on it. If you have RStudio, that's something that you can do quite easily. If not, you can download it. But it works. So you need to instal the library first, the package and the libraries with causal impact. And then if you have the data, that's quite easy to input, because the first thing that you have to do, if you have for example, Google Search Console data, is just to prepare the data so it's good enough to be inputted in the model.
So you can see here I just put the date in, I think it's in descendant fashion. Anyway, the closer in time it is to me at the top you have the least recent date, let's say it like that. So it needs to go down in a descendant fashion. And then you take your variable of interest. On causal impact normally I just do one variable at a time. I'm sure that there are other scripts that allow you to do more variables at a time, but the one that I want to test first is the clicks. And the first column will always be the one that you want to obtain data on. The rest is controls. If you have controls, then you can put them here. But in general, these are the two columns that you want. Again, as we mentioned, the first column is always your test groups, and other columns can be used as control groups.
The pre-period. So whenever you prepare the data for causal impact, you should get enough data and that goes for other models as well. But you should cover enough data to allow the model to make its predictions. So at least twice the post-period. So if you've been running a test for two weeks, you want six weeks worth of data, because the model needs four weeks to make its predictions. And then your two weeks post-period will be when you actually want to run the check-in. The thing that I noticed here is that sometimes you will have isolated zeros in the dataset. And that's something that you can easily counteract by applying some corrections and borrowing one unit from the row below or above.
But if you have multiple zeros, then you want to leave that data set alone, because probably you have not enough traffic, or not enough data to make some good predictions, and then your test is not going to be reliable. And here is when I want to run my actual test, I can just input my clean data set. I know it's a bit small here, but you can see just an understanding of what I do. And in the environment I will have my data set. And then I go look for the date when the test went on. So I can see that the test went on on the 1st of April here, which is row 91. So this allows me to establish my pre and post-periods. So I say my pre-period is from row one to 90, and then my post-period will be from row 91 till the end of the dataset. So you can see that the pre-period is much longer than the post-period. And then I input this series of formula again, something that you can find easily in the guide, and I can put on later.
And then you plot the impact. And will show me that this test was actually a winner. So you can see here its effect. It brings also back a clear summary, so you can see by how much the impact was relevant, and then if it was statistically significant. And you can also do another thing, which is figuring out the control groups, so if the control groups were used at all, this only is valid if you have control groups. So there's a script. Again, in the interest of time, I'm going to fly through it, because you can find it in the guide. But a few things that I learned for testing from several trials and errors, I always bring this example on, because it's quite relevant to me. I love figure skating. And this was a debate that was happening between two Olympic champions. They were each bringing their own statistics to show which one was the best champion among them.
And that's when I figured out that statistics is great, but it's not infallible. And you need to counteract, and you need to use some critical thinking to make sure that you are not conveying something that is not representative of the truth. So make sure that you know that external events can impact your data and you take note of those. So Google algo updates of course, very relevant to us. Tools tracking failures, sometimes even our tracking tools can fail. And engineering releases that go out at the same time as your tests. Because obviously then it means that whatever you might observe on your pages might not all be due to your tests, but to other changes. So what I normally always recommend is to keep a living document where you make note of everything that goes on in the industry internally and holiday wise, so that you can always trace it back.
Then make sure that you counter for outliers if, this is an actual example, so if you notice the third panel, it's great. But if I look at the first two panels, you can tell that there's something that's not quite right. So this is an example of when outliers are muddling my data. So they cannot originate from new product launches within the test group. They can originate from holidays and seasonal events as we've seen. Or results only from one page. For example, if something goes viral and then everything comes back from that one page, then it cannot really be applicable to the rest of the dataset. And you can actually also get outliers from tracking bugs, because sometimes they will just zero out. But you can actually avoid them by applying some tiny changes.
So increasing the size of the test groups, for example, because it makes the test more reliable. Adding control groups when you can and pre-processing your raw data. So in this case, I didn't know that. I went back to processing my raw data when it was already too late and I had already done the test analysis. But you can tell here that there's something quite wrong, because from 1.7% or so of CTRs, I was suddenly getting 10% across four days only, and those were bots. So once I corrected for them, obviously I didn't get a winner, but I got something that was quite underwhelming. But still, that's the other challenge. Whenever we want really something to work and we push for a test, we want it to be a winner. But we need to make sure that we're not a victim of our own confirmation bias, because there is some tests that might look like this, and even when we feel strongly about them and when we feel like they will make a great impact.
So you can just, after you cried a little about them, you can either run the test a little longer or repeat them with bigger groups, because that will make them more reliable. However, if this doesn't change and it's still inconclusive or a loser, then it's probably best to revert the change and focus on other tests. So we made it to the final section. Again, I'm going to fly through it, because I am conscious of time, and I want to leave time for questions. But yeah, we don't have much left. So reporting. At this point, these are likely the kind of data sets that you're going to have and the visualisations that you have. But no one likes a lot of numbers without context.
They want to see a story behind it. They want to see what you did, did it go well? And what you can do with that. So there are some options to tell your stories. Something very simple is, for example, you can just put a trend line and superimpose your launch date, that already tells somewhat a story. But you can also build dashboards when you can in order to easily spot trends. And this helps, for example, when a stakeholder asks you, "So how's the test going?" And it's nowhere near your check-in dates. But you can also spot if something goes wrong in the meantime. So this is great if you use Looker Studio. But if you want to venture out of Looker Studio, this is a brilliant presentation by Gokce Yesilbas that came out on YouTube yesterday, and showed you how to tell a story with dashboards on Tableau. So if you feel like experimenting, this is a great tool to have.
So this is normally what I provide, when I run a test and I show it to my stakeholders, I normally put a summary together that shows hypotheses, groups and treatments launch and check-in dates, results and classifications. And these steps are mainly for me, because of replicability. I want to go back and see if I have another similar test, I want to be able to know what I did in this one. But the next steps is what is going to matter the most to your stakeholders? So make sure that you include that. This is sort of what I present. I got a template at the end of this presentation, so you can just access it and copy and paste it. But try to be concise and speak their language. Another thing that I normally do is to create my own repository of data to do yourself, your future self a favour really, because then you can already see what works best and what doesn't work. And this makes you more attuned to do strategic testing that creates better customer insights and allows you to nimble your strategy.
And after testing, what do you do? So I have my winners, I have my losers. The losers, I will rerun or revert. But if it's a winner, then you need to scale it, because it's potentially a great win for the business as a whole. The way that you prioritise projects, try to do it based on the effort and the value or impact expected. So anything on the right side of this plot, for example, that has high value and that has high value in general, you can either do at some point or now. And anything on the left side, it's what you're going to do later, or not do at all, because it's not a good use of your time. This is something that communicating priorities is something that us in bigger teams might struggle sometimes with, because we need to speak the language of the people that we work with and we need to convey how important a change is for us. So I recommend this article by Gospelogia on how to talk to your dev teams and how to organise your tickets for better impact.
So this is for example, something on my count of a Jira ticket when I have a winner test and I want to apply my changes at scale. So yeah, just try to speak to their language, the language of the stakeholders or your collaborators when you are presenting results and when you want to scale a change. And try to get them on board, try to get them excited about the results. Because once they are on board with your testing strategy, they will want to run more tests, and they will want to make even more of an impact on your business. If you don't have a team, or if you are not into sprints that allow you to make an impact, then try to force your way into those conversations, because fostering a culture of testing is really paramount, especially in these landscapes where everything is changing.
We need to figure out for our business what works best with our audience and with search engines. And that's it. So it was a dump of information on you all. And hopefully you found it helpful. If you have any questions, here's my template. I'm going to leave it on for a couple of seconds while I take questions, and when I leave the stage again to Jojo.
Jojo:
Thank you. That was fantastic, Giulia. I'm definitely going to have to watch this again and work through the slides I think, because there was so much value in there. I just want to make sure I properly digest it all. We do have a few minutes. Oh, and I loved the nineties theme by the way.
Giulia Panozzo:
Thank you.
Jojo:
Very happy to see Take That made their way in there. So we have got a couple of minutes left, a few minutes left for questions. Let me have a look. So Jandira has asked, suppose... Let me, I'll pop it on the stage. Suppose you were to use the international site e.g, brand.com versus brand.ie, as a control group. Would you also have to ensure the pages on the control in .ie are statistically significant to the variant in .com?
Giulia Panozzo:
So if I'm understanding this correctly. So the way that I understand statistical significance in this context is do I want to use an international market that's as good as the test group? Of course, I would want to make sure that I have enough traffic for each result to be significant. If on the other hand we're asking about test versus control significance, I think these will be already something that's looked into your test group results. So essentially when we look at control groups, if we're doing this with a causal impact for example, you have an understanding of how much that control was used. When we saw the columns, the white and black columns, that tells us if the control was used at all. The closer it is to one, the more likely it has been used by the model to establish the result or the impact.
However, yes, if we are only using like the international example, brand.com versus brand.ie, I want brand.ie to have at least, I mean, I don't want the same traffic or like I don't need the same traffic as the main brand, but I will definitely need enough traffic for it to bring any results at all. I'm not sure what that would be, because that's something that you probably need to establish based on your own traffic and your own test. But definitely the control is something that needs to be the closest you can, at least in trends to the test.
Jojo:
Okay. All right, brilliant. Thank you for that. And Simon has asked, this is a good question. How do you determine what to test, and what do you consider a quick win when it comes to running a test?
Giulia Panozzo:
So in general, I think maybe, again, it depends on who you work for and what the priorities are at business. I feel like especially in the current landscape, I tend to suggest what is most likely to make an impact on that, for example, on what's dropping. Like if I know the CTR is dropping, I'm like, "Okay, well what can make a quick win?" And I look at literature, I look at other websites. So if I know that CTRs are dropping, okay, is there anything on the product pages that I can add that will make CTRs best? For example, reviews is a quick win. Returns is a quick win that's based on ecommerce and marketplaces. But I feel like a lot of the time I test to avoid a loss rather than to actually implement something that's a mega win. But that's my experience. I'm sure that for other people it will be probably coming from a place where they see other competitors doing something that stands out, and that can be something they can test.
Jojo:
Okay. That's interesting. Yeah, proactive testing rather than reactive testing.
Giulia Panozzo:
Yeah.
Jojo:
Okay, cool. We did have another question, but I think it's going to be too complicated to answer right now. So I've commented for Pradeep. Maybe he can audit, use Sitebulb to do an audit of the website that he's asking about. But that was really fantastic, Giulia. We're pretty much at time. So thank you all for coming. You will be the first to hear about any upcoming free trainings that we do and webinars via email. Our April webinar is going to be a panel discussion on marketplace SEO with two powerhouse SEOs from massive online marketplaces, Zoopla and apartments.com. So if you're an SEO managing a large marketplace or listings website, this is going to be the webinar for you. Keep an eye out on our socials for the announcement that registrations are open for that one. And people are saying lots of lovely feedback in the chat. Hopefully you can see that now, Giulia. Awesome. Amazing. Brilliant. Incredible. So thank you again from all of us at Sitebulb. Goodbye, and hope to see you all again soon. Goodbye.
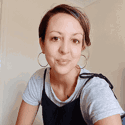
Jojo is Marketing Manager at Sitebulb. She has 15 years' experience in content and SEO, with 10 of those agency-side. Jojo works closely with the SEO community, collaborating on webinars, articles, and training content that helps to upskill SEOs.
When Jojo isn’t wrestling with content, you can find her trudging through fields with her King Charles Cavalier.
Articles for every stage in your SEO journey. Jump on board.
Related Articles
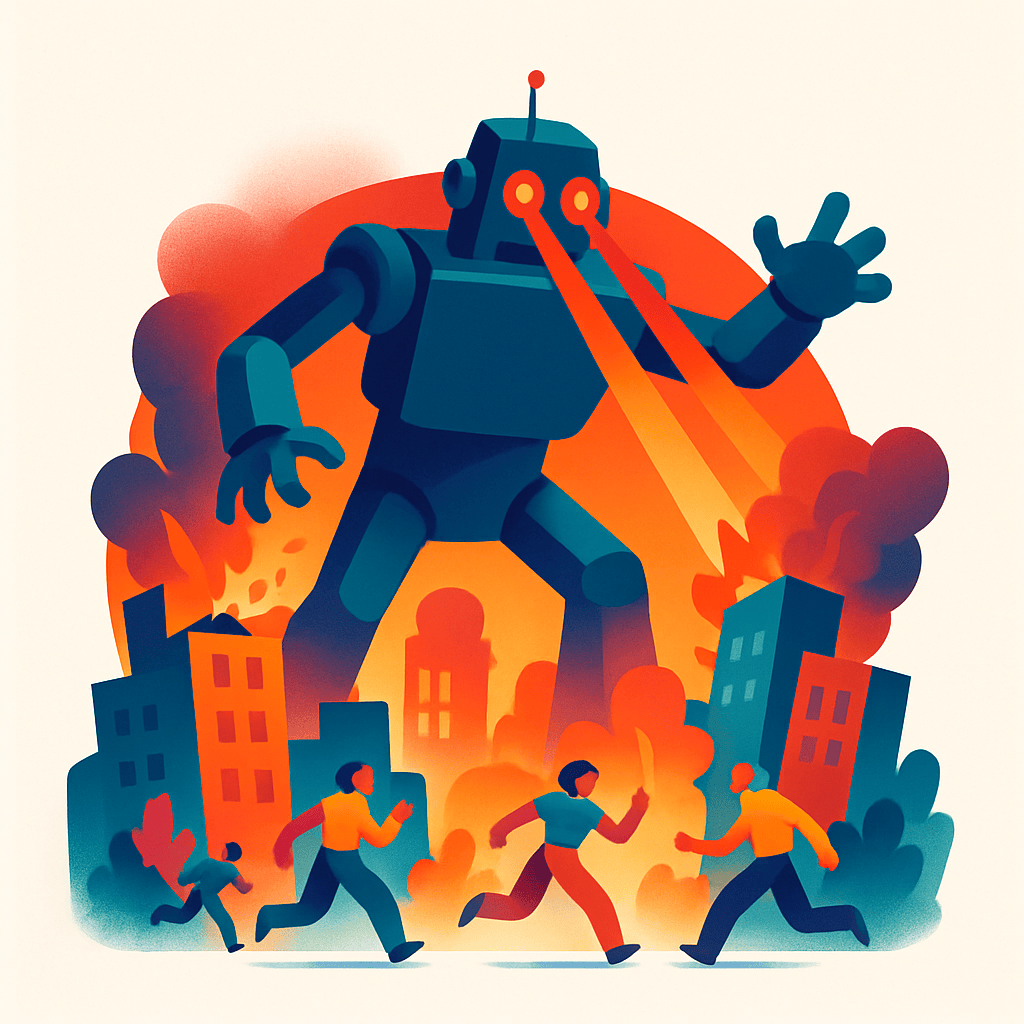
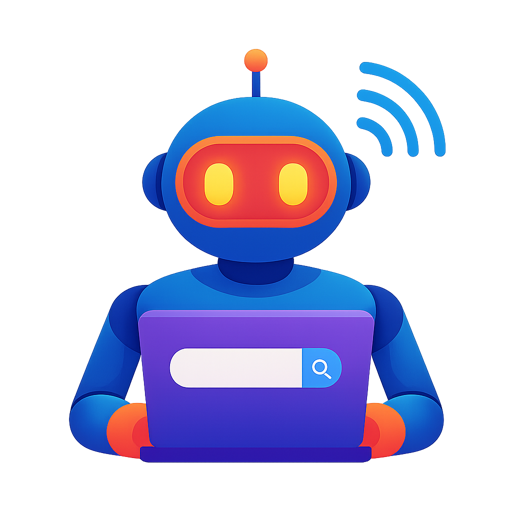
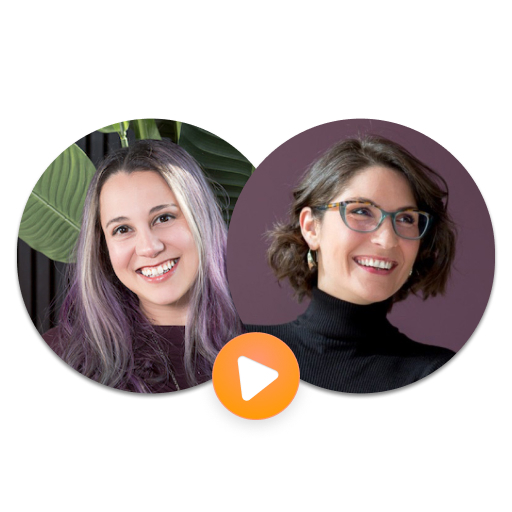
Sitebulb Desktop
Find, fix and communicate technical issues with easy visuals, in-depth insights, & prioritized recommendations across 300+ SEO issues.
- Ideal for SEO professionals, consultants & marketing agencies.
Try our fully featured 14 day trial. No credit card required.
Try Sitebulb for free
Sitebulb Cloud
Get all the capability of Sitebulb Desktop, accessible via your web browser. Crawl at scale without project, crawl credit, or machine limits.
- Perfect for collaboration, remote teams & extreme scale.
If you’re using another cloud crawler, you will definitely save money with Sitebulb.
Explore Sitebulb Cloud